Why we created the IP similarity feature
While we at GreyNoise have been collecting, analyzing, and labeling internet background noise, we have come to identify patterns among scanners and background noise traffic. Often we’ll see a group of IPs that have the same User-Agent or are sending payloads to the same web path, even though they are coming from different geo-locations. Or, we might see a group that uses the same OS and scanned all the same ports, but they have different rDNS lookups. Or any other combination of very similar behaviors with slight differences that show some version of distributed or obfuscated coordination.
With our new IP Similarity feature, we hope to enable anyone to easily sniff out these groups without having an analyst pore over all the raw data to find combinations of similar and dissimilar information. Stay tuned for an in-depth blog covering how we made this unique capability a reality, but for now, here’s a quick snapshot of what the feature does and the use cases it addresses.
The GreyNoise dataset
GreyNoise has a very rich dataset with a ton of features. For IP Similarity we are using a combination of relatively static IP-centric features, things we can derive just from knowing what IP the traffic is coming from or their connection metadata, and more dynamic behavioral features, things we see inside the traffic from that IP. These features are:
IP Centric
- VPN
- Tor
- rDNS
- OS
- JA3 Hash
- HASSH
Behavioral
- Bot
- Spoofable
- Web Paths
- User-Agents
- Mass scanner
- Ports
Of note, for this analysis we do not use GreyNoise-defined Tags, Actors, or Malicious/Benign/Unknown status, as these would bias our results based on our own derived information.
What GreyNoise analysis can show you
The output of the IP Similarity feature has been pretty phenomenal, which is why we’re so excited to preview it.
We can take a single IP from our friends at Shodan.io, https://viz.greynoise.io/ip-similarity/89.248.172.16, and return 19 (at the time of writing) other IPs from Shodan,
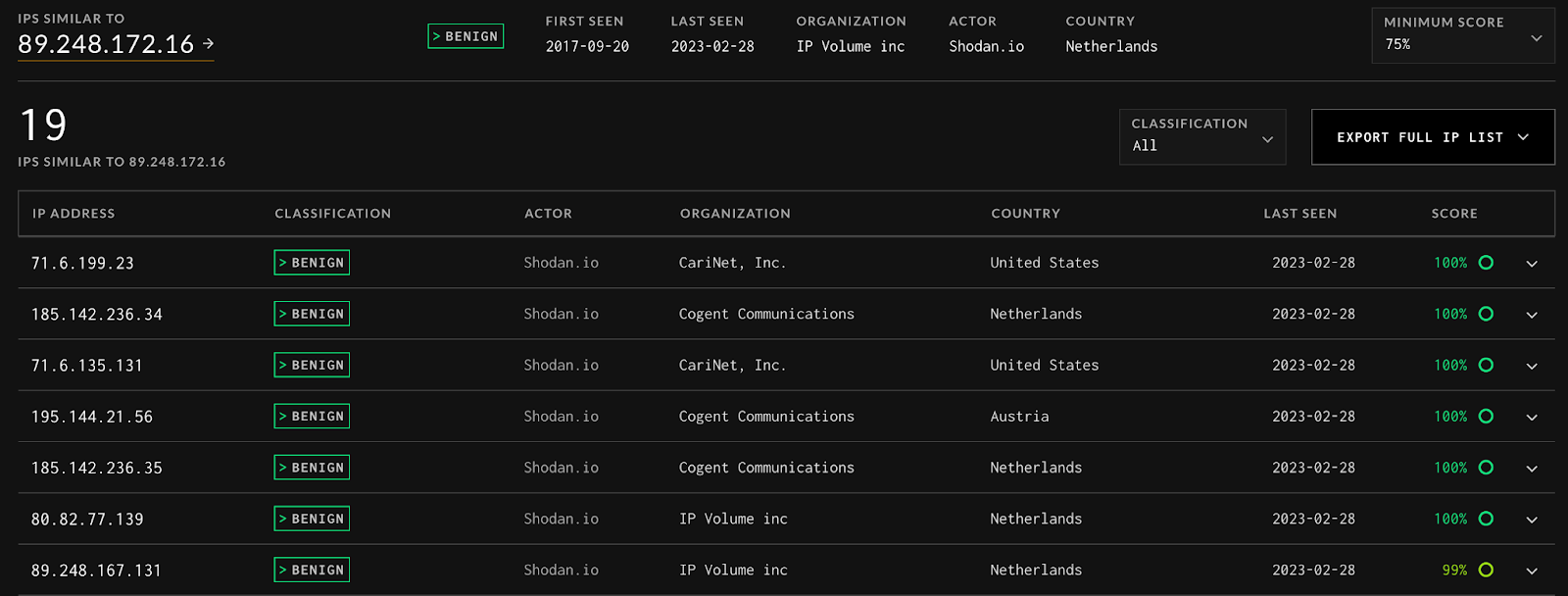
And we can compare the IPs side by side to find out why they were scored as similar.
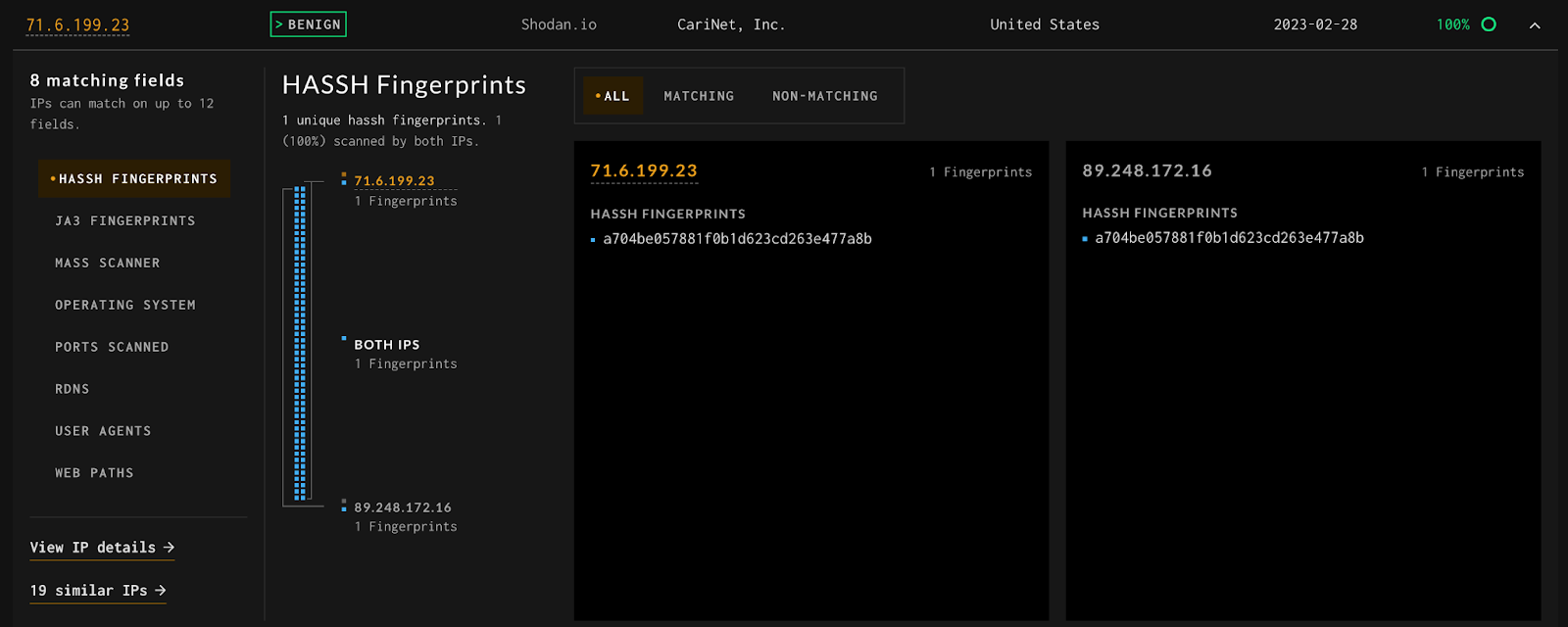
While we have an Actor tag for Shodan which allows us to see that all of these are correct, IP Similarity would have picked these out even if they were not tagged by GreyNoise.
Key use cases
As with any machine learning application, the results of IP Similarity will need to be verified by an aware observer, but this new feature holds a lot of promise for allowing GreyNoise users to automatically find new and interesting things related to their investigations. In fact, we see some immediate use cases for IP Similarity to help accelerate and close investigations faster, with increased accuracy, and provide required justifications before acting on the intelligence. For example:
- For cyber threat intelligence analysts. Use IP Similarity to generate a list of IP addresses that are similar to a target IP address identified/associated with specific malicious activity. This could entail generating a list of IPs similar to an IP observed executing a brute force attack.
- For threat hunters: Use IP Similarity to identify a list of IP addresses similar to your “hypothesis” IP address. You can then search for them inside your network (perhaps within your Splunk SIEM or leveraging NetFlow data). The goal here would be to proactively find compromises from related threat actors.
Get Access to IP Similarity
For more on how you can use IP similarity in your investigations, check out our recent blog from Nick Roy covering use cases of IP similarity. You can also read more about IP similarity in our documentation. IP Similarity is available as an add on to our paid GreyNoise packages and to all VIP users. If you’re interested in testing these features, sign up for a free trial account today!*
(*Create a free GreyNoise account to begin your enterprise trial. Activation button is on your Account Plan Details page.)

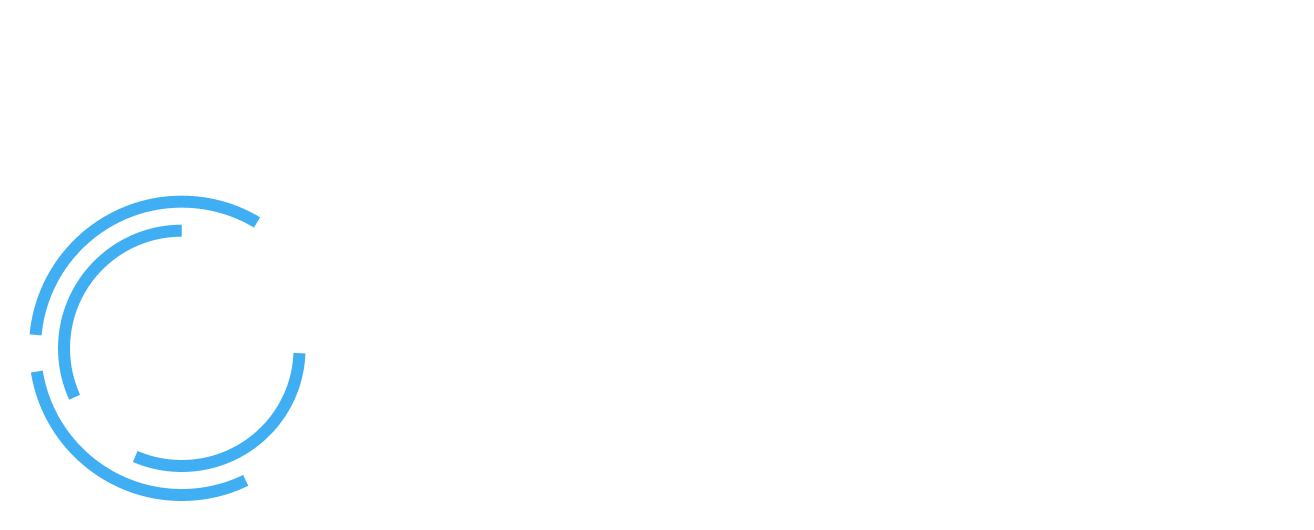